The latest buzz about Panama Papers has shaken the world. As we all know the Panama Papers is a set of 2.6 TB of data that includes 11.5 million confidential documents with detailed information about more than 214,000 offshore companies listed by the Panamanian corporate service provider Mossack Fonseca.
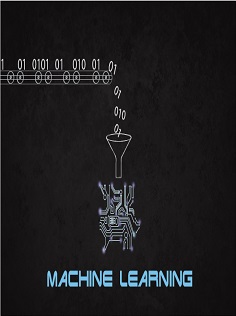
Business transformation with big data and machine learning
Webinar agenda
- Overview: Big data and machine learning
- Real world uses and benefits of ML
- Business uses of ML
- ML solutions for varied industries
- ML & data visualization: Seeing is believing
- Pathway to success – Onboarding (PoV)
- Demo
- Q&A
The Panama Papers has set an excellent example for the world about the importance of data science when it comes to analyzing big data. This leak makes us realize that appropriate approaches are needed to handle the challenges of data management for the present and the future.
Let’s take a deep dive into the Panama Papers and dig down the secret behind the biggest leak ever
This leak contains 4.8 million emails, 3 million database entries, 21.5 million PDFs, around one million images and 320,000 text documents. This is described as world’s biggest cache of data ever handed over to journalists.
How Süddeutsche Zeitung and ICIJ analyzed this data
Overall this leaked database has been analyzed with the use of latest techniques for advanced document and data analysis. This indicates the importance of technology’s role in helping the International Consortium of Investigative Journalists (ICIJ) and Süddeutsche Zeitung in creating the biggest news story of the year so far.
The main challenge in this scenario would be the variety of information that is provided to Süddeutsche Zeitung and the ICIJ. Most of the data they have received was unstructured data as we have seen in previous diagram. The most challenging aspect of analyzing data from heterogeneous sources is ingesting everything into a form that is consumable, queryable, and searchable. The indexing phase required for fast retrieval from massive unstructured data sources requires robust parallel processing as given below.
Let’s understand this process in detail. In its simplest form, unstructured data is in the form of text files and HTML files. It is relatively easy for engines to handle them as they are basically plain text.
Common document formats are only slightly more complex. Word processor documents, presentations, and so on mainly contain text but they may also have metadata and embedded content. They are popular and are not much more difficult to index compared to plain text files.
Container-like structures embed many objects along with their metadata. Folders and compressed archive files are some examples of container type formats. With some engines it is difficult to extract items inside containers and index them.
Larger and more complex container types such as Microsoft SharePoint, CMSs, and email archives have considerably more embedded items. Often, embedded components and metadata are stored separately. This kind of architecture does not lend itself to easy indexing. Therefore, despite availability of native searching capabilities, these systems are far more difficult to index for most engines.
The highest level of complexity is introduced by compliance-based storage systems. These are secure and often add a layer of obfuscation to make it difficult to tamper with data once it is stored in these systems.
In addition to the progressive difficulty of penetrating these various formats, there is the challenge of processing at scale, graceful handling of faults and failures, and load balancing. All this necessitates a specialized system that can perform these non-trivial tasks and transform unstructured data into a fully indexed, readily searchable form.
OCR and Image Processing
Many files and documents are not available in a digital, textual format. When it comes to images and pdfs, it is the most difficult task to analyze the data. Especially when they need to identify what do those images and PDFs contain, the language, how the text connected together with other blocks of text.
For instance, a newspaper clipping containing vital information cannot be directly fed into a retrieval system. It must be first transformed into a digital format and then its text must somehow be extracted from it.
This is where OCR comes in. Optical Character Recognition, or OCR, is the technology that helps read text off images containing handwritten, typewritten, or printed text. It produces a text file that can be indexed by an indexing engine.
A good OCR system allows custom specification of font name, size, spacing, and so on, and can adjust to different aspect ratios and scales. This enables highly precise scanning of text off images and PDFs and is particularly useful for processing items containing printed text. Modern OCR systems might also employ machine learning techniques to achieve a high level of precision. The ICIJ contacted big data analytics firm Nuix to help make sense of the vast amount of information it had received. Let’s see Nuix’s role in revealing the Panama Papers.
Text analytics
Text analytics is a wide field with various applications.
Named entity recognition (NER) is a subtask of text analytics that refers to finding elements of text that refer to predefined categories such as names of people, places, organizations, and so on, or numerical values such as monetary values, quantities, percentages, etc.
Some modern NER systems for English achieve near-human performance. Apart from grammar-based techniques, statistical models and machine learning techniques often aid NER in achieving greater performance.
Graph database:
A graph database, also called a graph-oriented database is a database that uses graph structures for semantic queries. A graph database is principally a collection of nodes and edges to store, map and query relationships. Most of the graph databases are NoSQL in nature and store their data in a key-value store or a document-oriented database. Each node stands for an entity (for example, a person or business) and each edge signifies a connection or relationship between two nodes. Every node in a graph database is defined by a unique identifier, a set of outgoing edges and/or incoming edges and a set of properties stated as key-value pairs. Graph databases are best suited for analyzing interconnections, that is the reason many Fortune 500 companies use graph databases to mine data from other sources.
How Gunnlaugsson hides his secret assets.
Graph databases are also beneficial for dealing with data in business disciplines that includes complex relationships, such as those in supply chain management, identification of the source of an IP telephony issue and serving recommendations based on the graph.
How graph database helped ICIJ
ICIJ had already demonstrated its ability to coordinate worldwide investigations numerous times in the past with the help of Linkurious Enterprise. Linkurious is a partner of the ICIJ since the Swiss Leaks scandal.
The challenging part for Linkurious Enterprise was to demonstrate data analysis proficiency to make the documents exploitable by the reporters. They pulled out the metadata of documents with the use of Apache Solr and Tika, then connected all the information together from the leaked databases, and created a graph of nodes and edges. The data was stored in the Neo4j graph database provided by their partner Neo Technology. After in-depth analysis, they got unique insights into the offshore banking sector indicating the relationships between banks, offshore companies, clients and their lawyers. In this case this graph includes many public personalities worldwide who have used Mossack Fonseca’s services. Let’s look at the example given below:
The network of middlemen and companies hiding Putin’s wealth
All the practices used by the journalists are very simple as they have collected lists of high profile individuals and their first circle. Then they have tried to identify links between these individuals and offshore entities and connected the dots together and quickly did the fact checking with the help of graph database technology.
Revealing the secret:
All of us know that the secret behind the leak of the Panama Papers is definitely the information the documents contain, but the real hero in this story is data science, which enabled the ICIJ and Süddeutsche Zeitung to carry out this journalistic scoop.
This is not the end of the revolution- the bigger picture is yet to be revealed.
Tip of the day: “If you have tons of data in various formats that needs to be analyzed, feel free to contact our data scientists.”
Special thanks to Shail Deliwala, Data Scientist at Softweb Solutions, for his technical inputs.