An individual today can unlock their phone by merely looking at it. This is a basic example of facial recognition powered by computer vision models. The ability of machines to “see” is transforming numerous fields. Businesses are leveraging computer vision solutions for various use cases like improved security, medical diagnoses, etc. However, without high-quality, well-annotated data, these models wouldn’t be nearly as effective.
The cost of bad data is an astonishing 15% to 25% of revenue for most companies. – MIT Sloan Management Review
To avoid such costs, it is essential to label visual data used to train computer vision models. This is where image annotation comes into the picture. It is a technique used to label digital images to describe the data features that a model should recognize. This blog highlights the basics of image annotation, its benefits, types and use cases.
What is image annotation?
Image annotation is a process of training computer vision models to produce more accurate results. We provide labels and descriptions to images, helping AI models learn and recognize specific objects, scenes, or concepts. This labelled data is the foundation for training powerful machine learning models.
Benefits of image annotation
- Accurate and reliable AI models: High-quality image annotation is the foundation for building powerful and trustworthy AI models. Precisely labelled data ensures that AI learns the right way. This is essential to build models that can make accurate decisions and perform tasks effectively.
- Cost-effective training for machine learning: Clear and accurate labels lead to a more efficient and affordable training process.
- Building intelligent machines: Image annotation is a cornerstone of creating powerful, reliable, and versatile AI models that can understand and interact with the visual world around us.
Suggested: AI-powered video analytics
What are some image annotation techniques
Bounding box annotation
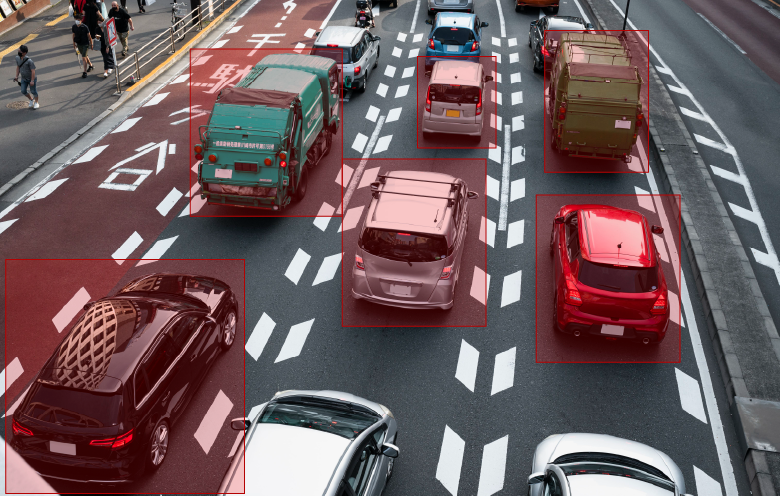
Bounding box annotation is a technique used to outline the boundaries of objects in an image or video, including their position and size. These bounding boxes help computer vision models learn the spatial features of the objects. This is important for accurate object detection in complex backgrounds or when there are multiple objects.
Why use bounding box annotation?
- Bounding boxes are intuitive and easy to understand.
- For many object detection applications, especially those involving common objects with relatively regular shapes, bounding boxes provide enough accuracy.
- Training AI models with bounding box annotations can be computationally less expensive compared to polygon annotations.
Polygon annotation
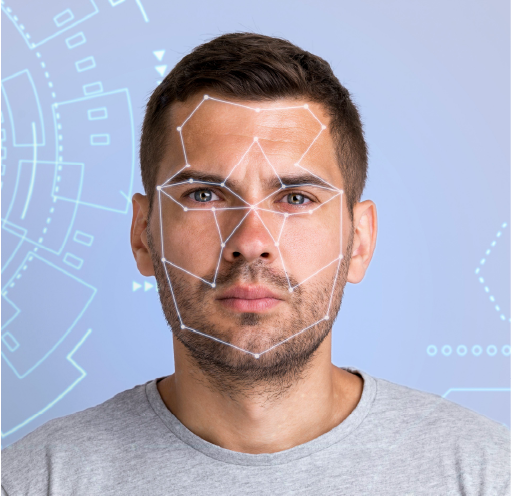
This type of image annotation uses a series of points to outline an object and capture its shape and boundaries. It connects a series of points to create a polygon that precisely follows the object’s boundaries.
Why use polygon annotation?
- Polygons excel at capturing the true form of objects with non-linear edges.
- By specifying numerous data points, polygon annotation achieves a high degree of accuracy, essential for scenarios requiring detailed labelling.
- Polygons focus solely on the object of interest, leading to cleaner data and potentially improving data augmentation techniques.
Keypoint annotation
It is an image labelling technique that identifies and marks important features in image or video. It focuses on specific, informative points on an object, rather than its entire outline.
Why use keypoint annotation?
- Keypoints pinpoint crucial locations like joints, extremities, or landmarks.
- Keypoints excel in tasks involving movement or pose analysis.
- Keypoints require labeling fewer data points.
- A single keypoint labeling scheme can be adapted to various objects by defining relevant keypoints for each.
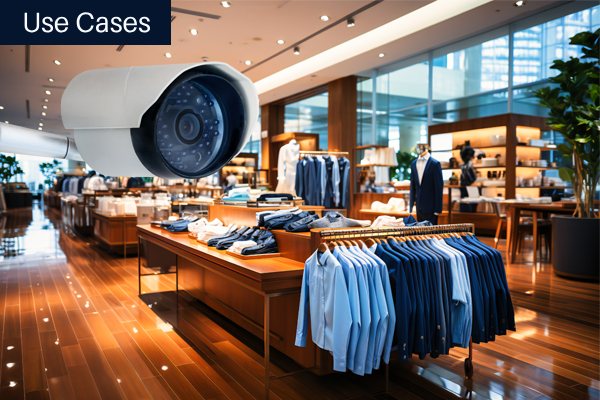
Let’s explore how CV is revolutionizing retail through:
- Smart shelf management
- Image recognition
- Targeted marketing
- AI-powered loss prevention
3D cuboid annotation
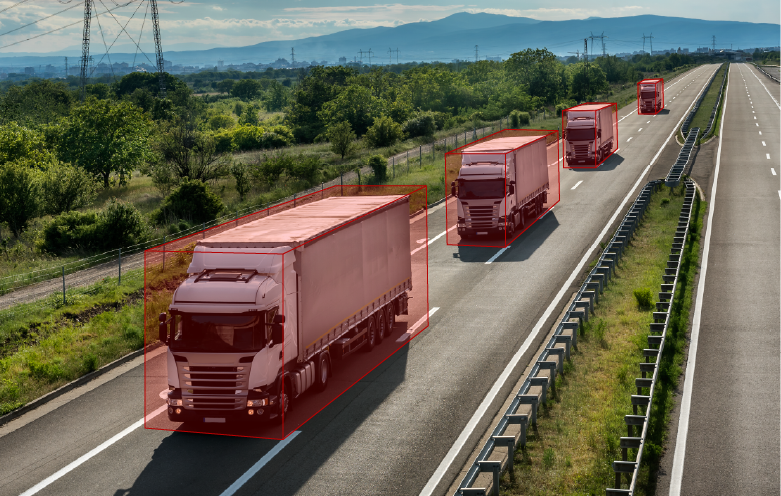
This technique involves creating a 3D simulated environment from 2D data. This allows businesses to grasp object’s size, position, and orientation in real-world space.
Why use 3D cuboid annotation?
- 3D cuboids add height.
- 3D cuboids can help AI models differentiate between similar objects in cluttered environments.
- Robust and transferable learning with more realistic 3D simulations for training AI models.
Semantic segmentation
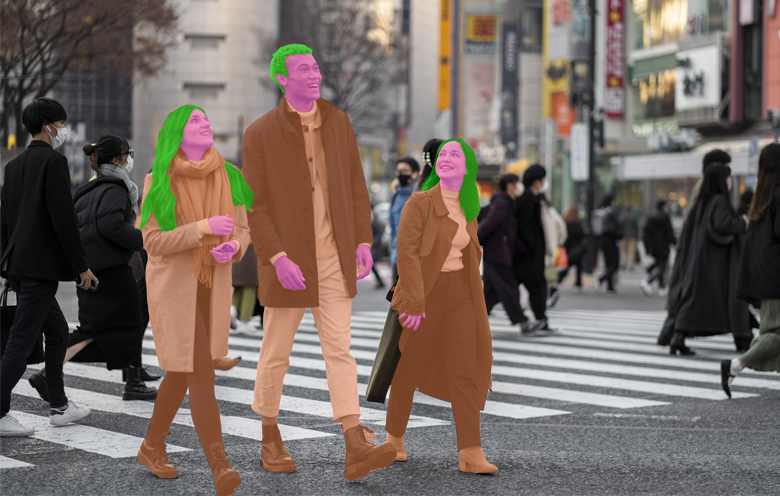
This computer vision technique assigns a class label to each pixel in an image, and then groups pixels with the same class together to create a segmentation mask. This is used for advanced scene understanding and analysis.
Why use semantic segmentation?
- Semantic segmentation allows the AI model to understand the exact contents of each image region, like differentiating between grass, sidewalk, and road in a street scene.
- By labeling each pixel, semantic segmentation provides a comprehensive breakdown of the image content.
- Semantic segmentation excels in scenarios with overlapping objects, cluttered scenes, or objects with irregular shapes.
- This technique is particularly useful for objects that are difficult or impractical to count individually, like grains of sand, leaves on a tree, or fur on an animal.
Image annotation use cases
Retail
Retailers can automatically track inventory levels on shelves by identifying and counting products in images. This allows for better stock management, targeted advertising, and improved customer service. Some of the examples of how retailers can use image annotation:
- Count the number of products in inventory, product line, and store shelves.
- Scan barcodes or QR codes on product labels or packaging materials.
- Evaluate the quality of thermal or heat seals on packaging.
Manufacturing
Annotated images can be used to train AI systems for automated visual inspection of products on assembly lines. This facilitates early detection of defects, leading to higher quality control and reduced production costs. Some of the examples of how manufacturers can use image annotation:
- Monitor and trace surgical instruments before and after surgical process.
- Medical images analyzed and classified for diagnosis and treatment.
- Monitor and track workplace safety with Vision AI real-time monitoring solutions.
- Determine the number of units or items in a package or container.
- Detect and categorize defects in pharmaceutical products, ensuring only high-quality items reach the market.
- Identify and address issues such as punctures, leaks, and irregularities in blister packaging for pharmaceutical products.
Healthcare
Medical imaging
Image annotation plays a vital role in medical diagnosis by training AI models to analyze X-rays, CT scans, and other medical images. This allows for faster and more accurate detection of abnormalities, leading to earlier intervention and improved patient outcomes.
Drug discovery
Annotated images of cells and tissues can be used to train AI models to identify potential drug targets and accelerate the development of new medications.
Traffic management
Annotated images help analyze traffic flow, identify congestion hotspots, and optimize traffic light patterns, leading to smoother commutes and improved road safety.
- Extract real time vehicle data for parking, tolls, and monitoring traffic.
- Identify vehicles traveling at an unsafe speed on the road.
- Get data on roadblock locations and optimize traffic flow.
- Automatically counts traffic such as vehicles, pedestrians, bicycles, etc.
- Improve transportation efficiency with vehicle tracking.
- Track vehicle lane conformity with vision AI.
Food and beverage
Automated quality control
Image annotation can train AI systems to inspect food and beverage items for defects like size discrepancies, discoloration, or foreign objects. This ensures consistent quality and minimizes waste.
Food safety monitoring
Annotated images can be used to train AI models to identify potential contaminants like mold or pests in food products. This proactive approach enhances food safety and consumer trust.
Satellite and aerial imagery
Urban planning
Optimizing city development by identifying suitable locations for infrastructure projects, green spaces, and residential areas.
Object detection and recognition
From identifying buildings and roads to monitoring ship traffic, image annotation empowers AI models to detect and recognize specific objects within aerial and satellite imagery.
- Optimize city development by identifying suitable locations for infrastructure projects, green spaces, and residential areas.
- Identify buildings and roads to monitoring ship traffic.
- Identify and locate potholes or road surface defects.
- Get live data on roadblock locations and optimize traffic flow.
- Detect the occurrence of accidents on the road.
Leverage image annotation services to ensure model accuracy
Image annotation stands as a pillar supporting the advancements in computer vision and artificial intelligence. Its applications span numerous industries, promising a future brimming with intelligent machines. As this field continues to evolve, staying informed about the latest image annotation techniques and best practices will be paramount.
Connect with our computer vision experts to better understand the technology and its benefits for your organization.