Sentiment analysis allows companies to extract insights from large pools of text data, such as customer opinions, social media posts, and news stories. It classifies opinions as positive, negative, or neutral using natural language processing, offering useful feedback about products, services, or brands.
In application, sentiment analysis relies on algorithms such as Naive Bayes, Support Vector Machines (SVM), and Recurrent Neural Networks (RNN). They assist in determining the overall sentiment and emotional tone expressed in the text.
Advanced AI techniques can achieve up to 85% accuracy in identifying sentiment polarity (positive, negative, neutral) – Marketing Scoop
In addition, NLP for sentiment analysis can be used in combination with other data analytics tools to give a full picture of customer conduct and market trends. By incorporating sentiment data into demographic data, buying history, and browsing habits, companies can better understand customer preferences and adapt their strategies accordingly. An integrated approach here improves customer satisfaction but also fuels growth and innovation.
Elevate your business with advanced NLP solutions
Tap into the full potential of natural language processing to enhance customer experiences and drive innovation. Let our expert team take your business to new heights.
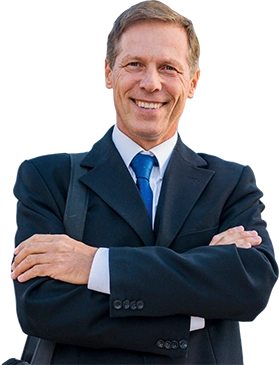
What is sentiment analysis in NLP?
Sentiment analysis with NLP is a technique that detects the emotional condition or sentiment of a situation, usually employing NLP to process text data. Language acts as an intermediary for human communication, and every statement has a sentiment, which may be positive, negative, or neutral.
For instance: There is a beverage chain business that sells different types of beverages such as milk shakes, iced teas, lemonades, mocktails, and soft drinks. They have developed a website where customers can place orders for drinks and leave reviews.
Reviews received:
Through the analysis of these reviews, the firm can conclude that they must emphasize advertising their milk shakes as well as enhancing the quality of their iced tea to raise overall sales.
Why is sentiment analysis important for businesses?
NLP-powered sentiment analysis is important for several reasons:
- Business intelligence: It assists businesses in knowing how their customers feel about their services or products. This can inform improvements, resolve customer issues, and increase overall customer satisfaction.
- Market research: Through sentiment analysis of how people feel about products, services, or brand mentions on social media, businesses can obtain information on market trends and competitors.
- Customer service: Sentiment analysis can be used to detect negative reviews or complaints in real-time, enabling faster response and fixing of problems.
- Product analytics: It can be employed to learn about user opinions regarding different aspects of a product, facilitating product strategy and development.
- Public relations: Sentiment analysis can be employed to track public opinion about a company or an individual, allowing for proactive public relations handling.
5 types of sentiment analysis
1. Fine-grained sentiment analysis
A lexical method is employed in fine-grained analysis to go deeper into the sentiments expressed in a provided text, while aspect-based sentiment analysis focuses on specific textual aspects. Depending on intensity, emotions, and objectives, it helps identify more accurate sentiments.
2. Emotion detection
The purpose of this type of NLP activity is to locate information about the emotions portrayed in a certain piece of text. Because it is intended to understand the emotional state of the author, it is more intricate than standard sentiment analysis. It can identify feelings such as surprise, fear, anger, and pleasure with finesse.
3. Aspect-based sentiment analysis
The objective of this NLP for sentiment analysis is to find and pick out opinions regarding specific aspects of a given text. It is a deeper type of classical sentiment analysis where the common feeling on a given topic is labeled as being favorable, negative, or neutral.
4. Intent-based analysis
This sentiment analysis in NLP applies machine learning algorithms to understand the text’s inherent intent aside from finding the text’s tone (negative, positive, or neutral). This helps to understand whether the text is issuing an order, expressing a want, complaining, or inquiring. In addition, it identifies the text’s sentiment and to what degree it is correct.
5. Multilingual sentiment analysis
Sentiment analysis across languages can be challenging. It takes a great deal of resources and preprocessing. Most of these resources – like sentiment lexicons can be found online, but some like translated corpora or noise detection algorithms need to be made. To use them, you’ll need to be proficient in coding. Machine learning services help train a sentiment analysis model to label texts in your preferred language. Alternatively, you can use a language classifier to automatically detect language in text.
How is NLP used in sentiment analysis?
Having understood what sentiment analysis in NLP is and its types, let’s explore how NLP is used for sentiment analysis.
1. Text preprocessing
NLP starts with the preprocessing of raw text data. This includes text cleaning and preparation, which usually comprises:
- Tokenization: Tokenizing the text into words, phrases, or tokens.
- Stemming: Cutting down words to their base form (e.g., “running” becomes “run”).
- Stop word removal: Removing unimportant words such as “the,” “and,” or “in” which do not possess important sentiment data.
2. Feature extraction
After preprocessing the text, NLP methods are employed to derive meaningful features from the text. These features may be:
- Keywords: Identifying words or phrases that express sentiment (e.g., “love,” “hate,” “excellent”).
- Named entities: Identifying entities like product names, brand names, or individuals, which can influence sentiment.
3. Sentiment classification
NLP models, frequently machine learning-based, are trained on labeled data to classify text as belonging to certain sentiment classes. Typical sentiment classes as we have seen in the beginning, are:
- Positive: Suggests a positive attitude or opinion
- Negative: Expresses a negative sentiment or opinion
- Neutral: Shows a lack of strong feeling, typically used for factual statements
4. Sentiment scoring
Sentiment analysis software provides a sentiment score or polarity to the text. This score measures the expressed sentiment as a number that reflects the intensity of the sentiment. Scores may vary between -1 (negative) and 1 (positive), with 0 generally being neutral.
5. Aspect-based sentiment analysis
As discussed above, NLP, besides document-level sentiment analysis, may conduct aspect-based sentiment analysis as well. This approach captures sentiments not only at the document level but also at the level of individual aspects or features in the text. For instance, in product feedback, it is able to separate sentiments regarding different product features such as design, performance, or price.
6. Contextual understanding
NLP methods allow sentiment analysis software to take into account the context and subtleties of language, enhancing accuracy in sentiment categorization. Recognizing sarcasm, irony, or sentiment shifts in a text is important for proper analysis.
7. Real-time insights
Organizations receive real-time insights regarding customer sentiments and market trends from NLP-based sentiment analysis. Companies are able to react to developing problems or take advantage of good vibes on a timely basis.
Applications of NLP-based sentiment analysis
Area | Application |
---|---|
Customer feedback | Companies employ sentiment analysis to analyze customer feedback and reviews. This assists them in understanding customer satisfaction and preference and making informed decisions. |
Social media monitoring | Companies track social media sites to know what people think about their product or service. This can be helpful in reputation management and also in detecting possible crises before they become full-blown crises. |
Market research | Sentiment analysis can be applied to know the public opinion regarding a product or an event. This can be helpful in market research. |
Product analytics | Sentiment analysis is employed by companies to collect insights from product reviews. This can inform product improvements and innovations. |
Healthcare | In the healthcare sector, sentiment analysis can be applied to collect knowledge regarding patient experiences and opinions regarding treatments, physicians, or hospitals. |
Finance | In finance, sentiment analysis is employed to measure sentiment in the marketplace. Market sentiment is used by traders and investors to make decisions. |
Human resources | Sentiment analysis with the aid of NLP is employed by HR departments to comprehend feedback from employees and enhance the work environment. |
How businesses use sentiment analysis to predict trends
Research and analysis of market trends
Sentimental analysis tools dissect a brand, product, or feature’s web presence to know market trends. It can similarly be utilized by a new brand to learn industry trends and assumptions particular to its market. The business can use this data to guide crucial business decisions regarding new product launches, features, and other issues.
Deep dive analysis algorithms have enabled companies to comprehend features, attributes, and customer opinions about a product. These findings help businesses tailor and appeal to the target audience with their offerings.
Catering to customer service
Customer service management is a challenging task owing to the large number of requests, diversity of topics, multiplicity of corporate departments, and immediacy of any specific request. A great deal of customer information is generated through customer support exchanges, including chat logs, voice recordings, product reviews, and emails that are cast into an NLP technology model.
This model uses the data to do sentiment analysis and generate a response. This answer can help identify the priority levels of the customer’s choices and the most suitable resource for a variety of questions.
Decision-making for investment
Stock price movements are significantly affected by the high volatility of the financial market and psychological influences, including user perceptions of new investments, regulatory actions, or natural catastrophes. In a situation otherwise uncertain, examination of such information and financial news may assist in predicting successful outcomes.
To execute trades in millisecond steps, traders need lightning-quick reflexes. The attitude of the market is shaped by numerous factors. A correlation has been observed between the movement of a firm’s stock price and the polarity of the most frequently cited comments that contain its stock symbol.
Business intelligence
You can measure how potential customers perceive your brand through the use of sentiment analysis methods. Through analyzing social media and survey data, you can get essential information on how well your business is performing for your customers. Firms measure the target audience’s response to the service products.
Sentiment analysis NLP leverages huge amounts of online data and artificial intelligence-based technologies to understand the implied meaning in the text. By applying aspect-based sentiment analysis, businesses can:
- Maintain control over their brand reputation
- Discern gaps in their marketing strategy
- Focus on key areas where client attitudes are either positive or negative
Employee satisfaction
You can obtain information regarding employee behavior and manage their problems effectively by going through the questionnaires, peer ratings, and manager feedback. Even keeping track of employee dissatisfaction, demotivation, and possible behaviors to initiate activities that would make them feel productive can be helpful. Analyzing employee feedback tone can lead to enhanced output, attrition reduction, and employee engagement.
Marketing campaigns
Sentiment analysis software is applied in psychology, sociology, and political science for analyzing patterns, perspectives, inherent bias, quantifying response, etc. The characteristics of sentiment analysis can prove to be extremely helpful in creating marketing campaigns for product launches or for advertising corporate attributes or products.
You can concentrate on increasing audience engagement and contextualizing and adjusting important performance indicators. By considering the views of the clients, you can develop a better message for advertising and marketing efforts, which will enable smooth transitions.
Banking
AI and machine learning-driven sentiment research is essential for financial institutions undergoing a digital transformation to effectively sell financial products and services. Over the last decade, the financial sector has undergone a change. With the new innovative digital financial platforms and solutions, the new competitive start-ups have entered the market.
This is concerning for a business that is entrenched in its traditional practices. This requires NLP sentiment analysis to stay competitive and adapt to the evolving market landscape.
The techniques of sentiment classification
Traditional machine learning algorithms
- Naive Bayes: From the frequency of words or other attributes in the document, Naive Bayes calculates the probability that a document is of a specific sentiment class. It is computationally efficient and assumes independence of features.
- Support Vector Machines (SVM): SVM is a supervised learning method that separates data into different sentiment classes by finding an optimal hyperplane. SVM finds the maximum margin between classes with the high-dimensional feature space of the data.
- Random Forests: Random Forests predict emotion with an ensemble of decision trees. The ultimate prediction is made using the majority vote of all decision trees, and each tree is built independently. High-dimensional data can be accommodated, and complex relationships can be modeled through the assistance of random forests.
Deep learning models
- Convolutional Neural Networks (CNN): While their primary use is for image analysis, CNNs are also applicable to text classification issues. Convolutional layers allow CNNs to learn features automatically by detecting local patterns and hierarchical representations in the text data.
- Recurrent Neural Networks (RNNs): RNNs can well represent temporal relationships between text and work effectively for sequential data. RNNs analyze text by retaining a hidden state that stores data from previous words, which enables them to capture contextual data.
Architectures and approaches of machine learning and deep learning
- Transformer-based models: Transformer-based models, including BERT (Bidirectional Encoder Representations from Transformers), have achieved current state-of-the-art performance in sentiment classification. Transformer-based models use self-attention mechanisms to learn global dependencies and context-aware representations.
- Ensemble methods: These techniques combine multiple models to obtain a single prediction. This is achieved through averaging multiple models’ predictions or through training many models with different hyperparameters or topologies. Ensemble methods quite often make sentiment classification more robust and accurate.
Turn insights into action with sentiment analysis
Sentiment analysis helps you stay ahead in an increasingly data-driven world. By leveraging NLP to decode customer emotions, you can anticipate trends, enhance engagement, and refine your strategies with precision. From social media sentiment tracking to proactive brand management, this technology turns unstructured data into powerful, actionable insights.
As AI continues to evolve, businesses that embrace sentiment analysis will gain a distinct competitive edge. The real question isn’t whether to use it – but how quickly you can start transforming insights into impact. For more details on how sentiment analysis can help in the growth of your business, talk to our ML experts.