Did you know that only on average, 54% of AI projects make it from pilot to production?
While the potential of AI is undeniable, integrating it seamlessly into existing systems can be surprisingly challenging. In fact, 80% AI integration projects fail due to a combination of organizational hurdles and technical roadblocks.
Let’s explore five reasons why organizations fail in implementing AI in business processes and how to tackle these challenges to ensure successful AI integration.
#1 Lack of clear goal and strategy
“One of the biggest [reasons] is sometimes people think, all I need to do is throw money at a problem or put a technology in, and success comes out the other end, and that just doesn’t happen,” – Chris Chapo, SVP of data and analytics at GAP.
The reason this statement is so impactful is that AI projects require much more than just financial investment and technology deployment. Businesses need a thorough understanding of problem statement(s) that they want to address by implementing artificial intelligence.
With a comprehensive AI strategy in place, companies can realize the true value of AI within the organization. There are four pillars of a well-defined AI strategy that business leaders need to take care of:
- Vision: Align AI vision with business goals
- Value: Build a strong foundation with ethical and responsible AI
- Risks: Understand and mitigate broader risks
- Adoption: Start small and plan to scale up
Opt for a 10-20-70 formula as advised by Boston Consulting Group.
- 10% efforts to build machine learning models
- 20% efforts in maintaining high-quality data and technology adoption
- 70% efforts on developing new business processes or transforming the way business functions operate
Suggested: Is AI the key to your business’s success in the digital age?
#2 Poor data quality
“Data quality is directly linked to the quality of decision making,” says Melody Chien, Senior Director Analyst, Gartner. “Good quality data provides better leads, better understanding of customers and better customer relationships. Data quality is a competitive advantage that D&A leaders need to improve upon continuously.”
As per Gartner, every year, poor data quality costs organizations an average $12.9 million. Inaccurate, incomplete, and inconsistent data used to train AI models results in biased or ineffective algorithms. There are several consequences that can arise from using poor quality data for AI:
- Inaccurate results
- Bias
- Wasted resources
- Project shutdowns
- Reputational damage
To drive accurate insights for better decision making, it is imperative to use relevant and accurate datasets. Also, ensure that you utilize data from reliable sources that serve your business purpose.
Few of the important aspects to ensure data quality include:
- Data literacy: Foster data-driven culture to encourage employees to understand the importance of quality of data on organization’s overall performance.
- Data capture: Use automation tools to reduce manual data capturing error.
- Data governance: Implement data governance best practices tailored to your unique business case.
Here are 12 actions by Gartner that business leaders must take to improve data quality.
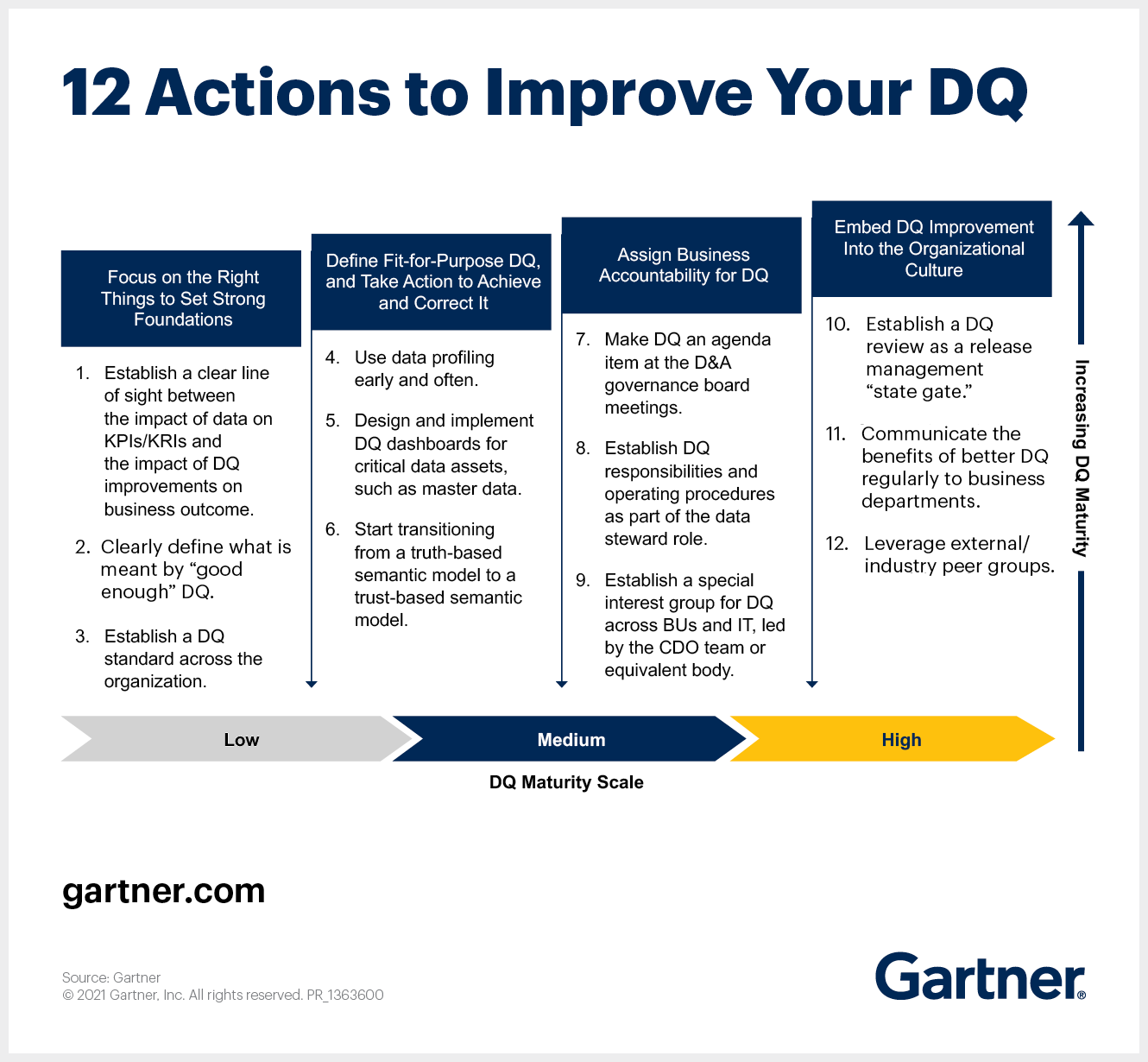
#3 Resource underestimation
“It’s [Implementing AI in business] not a one-person exercise,” says Chris Griffin, Audit and Assurance Managing Partner of Transformation and Technology at Deloitte.
It is crucial to understand that AI projects are resource intensive. AI implementation requires significant time, skilled personnel, and financial investment. Underestimating these factors can lead to businesses faltering to adopt the true potential of AI.
Leveraging AIOps in your project management practices helps you with improved capacity planning. AIOps refers to the practice of combining artificial intelligence into operations. It enables you to streamline processes and create a more proactive workforce. AIOps offers the following benefits:
- Allocate resources to ensure cost-effectiveness
- Proactive error detection
- Improved model efficiency
- IT spend reduction
- Better time management
McKinsey offers insights on how efficient resource allocation can increase your company values:
- Go granular: Test now, scale up later
- Focus on value creation: Understand that investments come in two flavors:
- Easy to value upfront (like building a new mine).
- Harder to value – their overall profitability over time is more important (like a business segment).
#4 Risk mitigation
“I think it’s promising that we have policymakers who are trying to get smart about this technology and get in front of risks before we’ve had mass deployment across the product space. I think there are some very obvious things that we need to establish, one of which is the right to know whether you’re consuming content from a bot or not.” — Clem Delangue, co-founder and CEO, Hugging Face
AI projects involve inherent risks, such as ethical considerations, bias in algorithms, and security vulnerabilities. Failing to identify and plan for these risks can lead to project failure. Risk mitigation involves identifying and addressing potential negative impacts of AI technology.
McKinsey & Company has crafted a framework that emphasizes on collaborative efforts to ensure that AI models align with social norms and legal requirements. This framework includes:
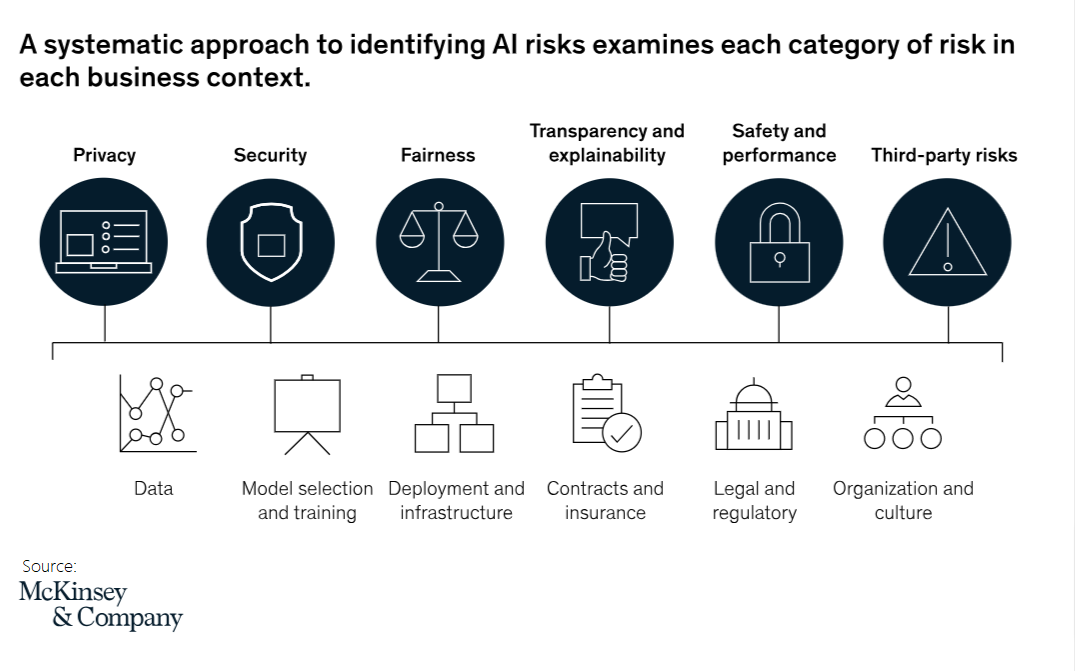
Focusing on results is important. However, it is equally important to manage potential AI-related risks, such as personal privacy and equity, and fairness, that high performing AI-adopters have a head-start on.
A strong responsible AI program acts as a shield, minimizing risks associated with AI development. It brings together policies, oversight, clear processes, and the right tools to ensure AI aligns with your company’s values and behaves ethically. Responsible AI reduces the risk of AI project failure by identifying and mitigating issues before the system fails.
#5 Vendor misalignment
IFS Chief Customer Officer, Michael Ouissi said. “Companies investing in technology should expect their vendors to adhere to sound sales and marketing practices based squarely in actual customer value.”
A recent IFS study found that 37% of business leaders noticed that poor vendor advice leads digital transformation projects to fail. In such cases, strong leadership and thorough understanding of the technology plays an important role. Here are a few steps for effective vendor selection and management:
- Create vendor profiles
- Evaluate domain expertise of the vendor
- Have an efficient project management plan in place
- Prepare vendor risk plans
- Monthly performance and contract review
- Risk review
Applying the five key findings from Deloitte’s executive Global Outsourcing Survey for AI vendor management:
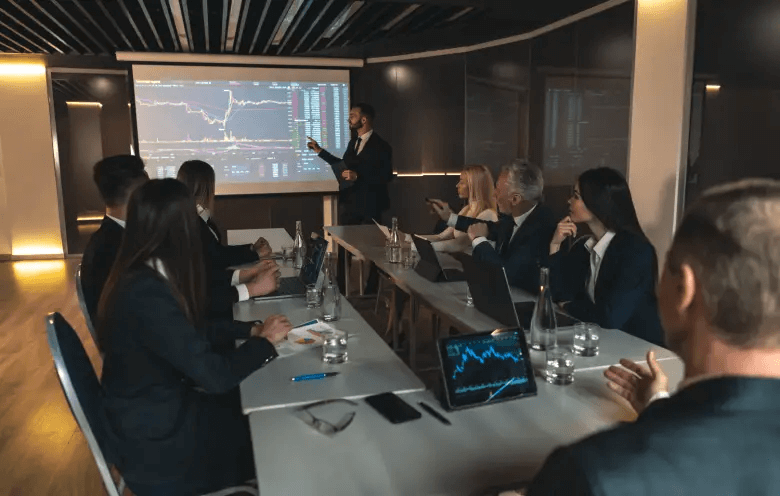
Building a winning AI strategy: A guide for CIOs
AI is transforming industries like manufacturing, hospitality, healthcare, and education. Don’t miss out on AI’s benefits—act now to learn more!
Right talent with the right skills at the right time
62% of executives say they are ill-prepared to address the causes and impacts of poor employee retention. This creates an opportunity for AI services providers to offer their in-depth expertise to address this skills gap problem.
Data security
81% of executives turn to third-party vendors to provide, in full or in part, their cybersecurity capabilities. While AI implementation provides multitude of benefits, there are security issues that need to be tackled too. Hence, it is imperative to leverage AI services from vendors who have robust data security measures to help you navigate risks.
Creating a holistic ecosystem
Enterprises need to have a robust framework to effectively manage an ecosystem of third-party relationship. Eliminate the traditional siloed vendor management approach and adopt business-led tech strategies.
Evolving third-party models
Leverage offerings by service providers that deliver knowledge, capability, and capacity. Ensure that they provide expertise that meet the changing market demands and needs. The key to maximizing the potential of the AI services offered by the IT vendor is to work in collaboration for better business value creation.
Build-Operate-Transfer-Transform
Businesses that lack the necessary capabilities can adopt this approach for their AI initiatives. Choose a vendor that helps you train your in-house team. Look beyond skillsets and consider time zones, cultural similarities, and enhanced infrastructure.
Leverage the true potential of artificial intelligence
Artificial intelligence holds immense potential, but its successful implementation requires careful planning and execution. By ensuring high-quality data, setting realistic goals, and having a clear strategic vision, organizations can leverage AI to achieve significant benefits.
Partnering with reliable third-party AI services providers can bridge skill gaps and provide access to cutting-edge tools, further increasing the chances of AI project success. Contact our AI consultants to get a better understanding of the benefits of AI and how to successfully implement AI in their business.