Data science is huge right now. It has become the Holy Grail for companies that want to discover insights from their existing data sets, and setup predictive analysis driven maintenance programs, and automate routine processes.
But to get good results from data science you require good data scientists; and therein lies the problem for many companies today. Under-qualified practitioners and promises of unbelievable productivity gains have started tarnishing the field. Some industry watchers view data scientists as snake oil salesmen while others see them as unicorns. This brings us to a quandary –
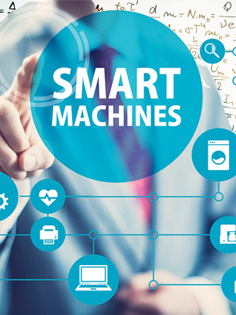
How AI and Data Science solve the biggest business challenges of today
Webinar agenda
- An Overview on Data Science and Artificial Intelligence
- Why every business needs Data Science and AI
- Business cases for varied industries
- Demo
- Roadmap for implementation
- Questions
Who is a data scientist? And how do you know if he is delivering value to your company?
There are several new organizations working on standardizing the field and the scope of work of a data scientist. But this problem is compounded by the fact that there is no standard definition of a data scientist and the programs offering degrees and certifications are new, few and relatively obscure.
In this blog post, we will cover the five things that every company should know before it decides to hire data scientists.
1. Your data scientist needs to be part mathematician, part business analyst and a good storyteller
A good data scientist will have completed at least a Master’s degree in mathematics, statistics, computer science or engineering. This is a requisite that cannot be compromised on since without a strong educational background, a data scientist won’t be able to use the right methodologies to get value out of your data. The second most important requirement is knowledge of analytical tools and programming languages such as SAS, R and Python. Other useful languages include Java, Perl and C/C++ along with a familiarity of Hadoop and other database systems.
Once you are sure of your data scientist’s technical skills, you need to find out his business skills. The difference between a data analyst and a data scientist is that while the former looks at just one source of data, e.g. a CRM or ERP system, the latter looks at data from all sources in the organization. This means that he needs to understand your company and the industry you are working in so that the insights he gleans from the data are practical.
Being able to communicate these insights in non-technical terms is the final and most important part of his job. This is where the storyteller part comes in. Decision makers in the marketing, sales and finance departments along with other C-level executives should be able to understand the reports he creates.
2. To get your unicorn, stop looking for a unicorn
This statement might seem contradictory, but trying to hire a data scientist based on how a few media publications portray the profession can only end up frustrating your search. A data scientist need not be someone with a PhD in mathematics and an MBA in finance from an Ivy League university along with extensive experience in handling Big Data.
Although a strong educational background is a prerequisite, an Ivy League degree is not. Nor does he need to know every BI program out there. A good data scientist will focus on the insights that can be gained from data instead of trying to master every analytic tool in the market.
Industry associations, conferences, online competitions such as the ones run by Kaggle are ideal hunting grounds when looking for your data scientist.
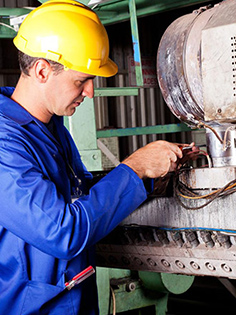
Predictive Maintenance in Manufacturing
Webinar agenda
- What is Predictive Maintenance
- Understanding of concept
- Benefits of Predictive Maintenance in manufacturing
- How to reduce costs with Predictive Intelligence
- Detailed insights on analytics
- Internet of Things platforms
- How IoT data is analyzed
3. Machines or humans, decide which one is the focus area of your data scientist
A data scientist usually focuses on one of the two areas – data science for machines or data science for humans.
If the aim of your data science project is to create automated programs that handle maintenance tasks, control product quality on the assembly line or create recommendation engines for your online store, then you need a data scientist focused on machines. This is because such data science projects require the creation of complex mathematical models based on machine learning, which involves algorithms. These algorithm-driven programs then need to act autonomously. The creation of such robust programs requires your data scientist to be extremely well-versed in both mathematics and computer science.
On the other hand, if your data science project is geared towards understanding user growth, predict market share and trends, then you need a data scientist focused on data analytics. He must be able to implement the data visualization tools in a manner that enables the decision makers in your company to understand the business value out of the data that is being generated.
4. Your data scientist is only as good as the data you give him
To get good results from your data sets, your data needs to be of the right kind and quality. Not all data is created equal. It could be the case that most of the data sets that you have are actually of little value.
For example, if you are trying to analyze the vibration patterns of your production line machines to improve product quality, then the previously recorded sensor data needs to be reliable. Faulty sensor data and inadequate data will result in inaccurate predictions. To ensure accurate results from your data, the first step is ensuring that it’s being gathered from clean sources. In a factory setting, this means ensuring all the sensors and equipment tags are working properly.
There are several other ways to ensure data integrity, including the use of statistical techniques, audit scripts that check for data that should exist but are missing, and the use of machine learning for data cleansing.
5. Abiding by a code of ethics
The data science team that you hire also needs to abide by a code of ethics. Ethical data scientists will not cherry pick data to produce results that management wants to see, but display them as they are, even if they show the company in an unfaltering light.
Correlation does not imply causation. This well-known phrase from statistics is widely used in data science circles to imply that just because there is a correlation between two factors does not imply that they are the cause of each other. Data scientists need to be aware of these, and other ethical dilemmas, to ensure that they do not build up false expectations in the client’s mind.
There are several other factors that you need to consider before launching a data science project in your company and hiring data scientists. For more information, you can talk to our data scientists on what it takes to have a successful Big Data project.
If you are looking for specific solutions in Analytics, Big Data, Data Mining and Data Science, you can also visit www.KDnuggets.com, which has extensive resources on these topics.